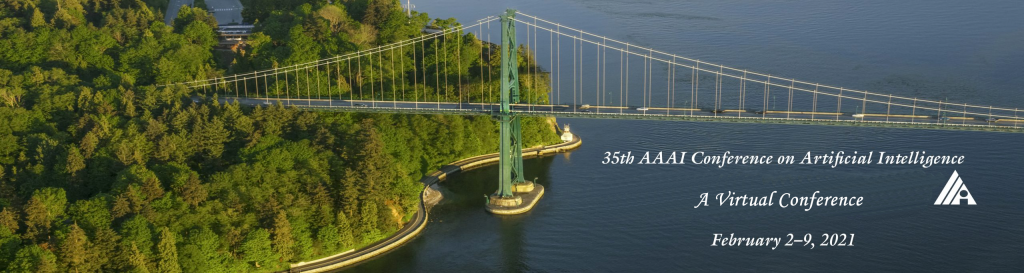
Our paper entitled “Non-asymptotic Convergence of Adam-type Reinforcement Learning Algorithms under Markovian Sampling” has been accepted to AAAI 2021. This paper provides the first such convergence analysis for two fundamental RL algorithms of policy gradient (PG) and temporal difference (TD) learning that incorporate AMSGrad updates (a standard alternative of Adam in theoretical analysis), referred to as PG-AMSGrad and TD-AMSGrad, respectively. Moreover, our analysis focuses on Markovian sampling for both algorithms. Convergence rates for PG-AMSGrad under general nonlinear function approximation with constant stepsize and diminishing stepsize are derived. Convergence rates for TD-AMSGrad under linear function approximation with constant and diminishing stepsizes are established as well. Our study develops new techniques for analyzing the Adam-type RL algorithms under Markovian sampling. Preprint is available at https://arxiv.org/pdf/2002.06286.pdf